Bioinformatics/ machine-learning
The integration of artificial intelligence (AI) into bioinformatics and materials discovery is progressively enhancing and expediting research endeavors. AI aids scientists in formulating hypotheses, crafting experiments, collecting and interpreting extensive datasets, and extracting insights that may have been unattainable solely through conventional scientific techniques. AI-driven approaches enable the rapid exploration and customization of biomaterial properties, aiding in the creation of innovative molecules with tailored characteristics for medical and biological purposes. The future promises more sophisticated AI algorithms and hybrid models that combine the strengths of AI and traditional multiscale calculations capable of making even more accurate predictions.
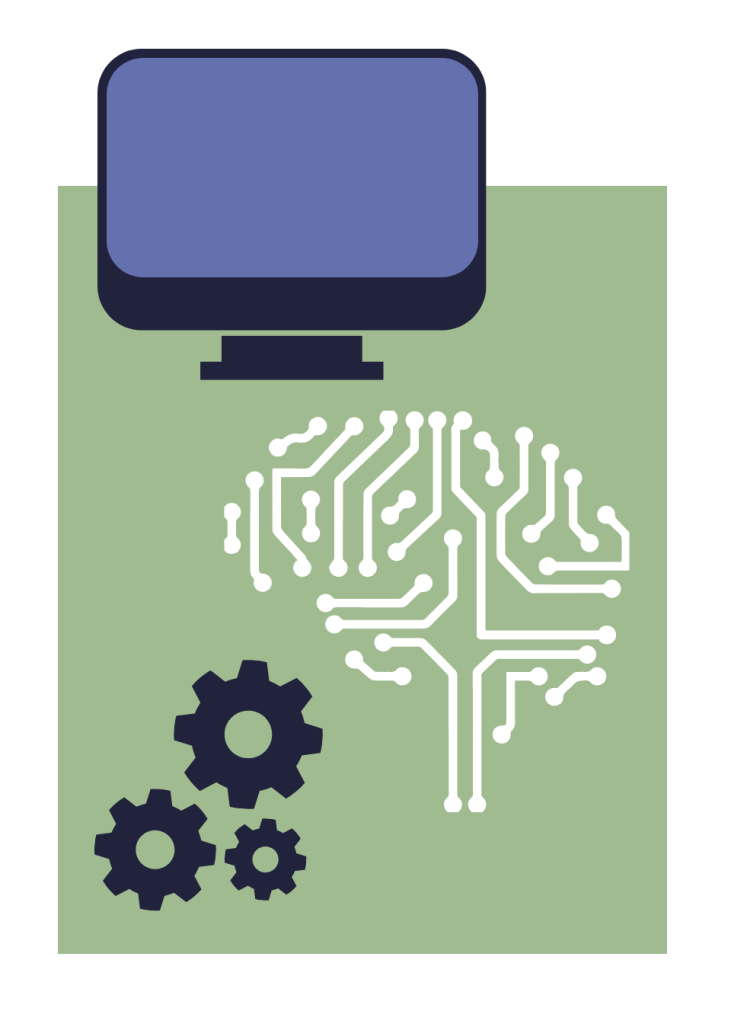
Publications
Frey, M.; Bathe, U.; Meink, L.; Balcke, G. U.; Schmidt, J.; Frolov, A.; Soboleva, A.; Hassanin, A.; Davari, M. D.; Frank, O.; et al. Combinatorial biosynthesis in yeast leads to over 200 diterpenoids. Metab Eng 2024, 82, 193-200. DOI: 10.1016/j.ymben.2024.02.006
Siedhoff N E, Illig A, Schwaneberg U, Davari MD. PyPEF-An Integrated Framework for Data-Driven Protein Engineering. J. Chem. Inf. Model. 2021, 61 (7) 3463–3476, https://doi.org/10.1021/acs.jcim.1c00099
Wittmund M, Cadet F, Davari MD. Learning epistasis and residue coevolution patterns: Current trends and future perspectives for advancing enzyme engineering。ACS Catal. 2022, 12, 22, 14243–14263. https://doi.org/10.1021/acscatal.2c01426
Siedhoff N E, Schwaneberg U, Davari MD. Machine learning-assisted enzyme engineering. Methods in Enzymology 2020, 643, 281-315 https://doi.org/10.1016/bs.mie.2020.05.005
Schindler B, Günzel D, Schmid T. Transcending Two-Path Impedance Spectroscopy with Machine Learning: A Computational Study on Modeling and Quantifying Electric Bipolarity of Epithelia. Int. J Adv. Life Sci. 2021, 13(1&2), 134-148, ISSN 1942-2660, http://www.iariajournals.org/life_sciences/
Collaborative research centers / Network
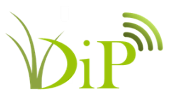